Organizers
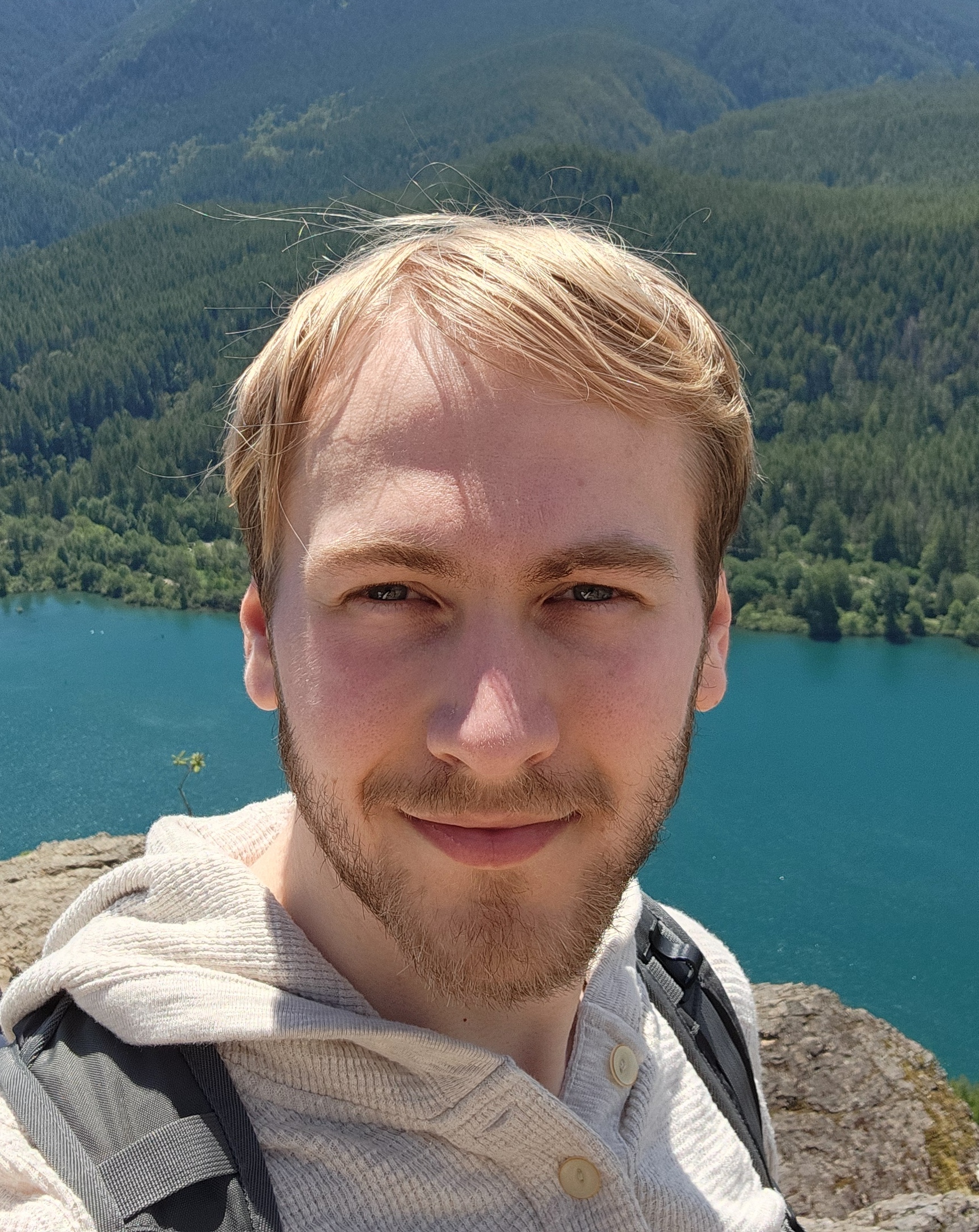
Nick Erickson is a Senior Applied Scientist at Amazon Web Services. He is the primary author of the open-source AutoML framework AutoGluon, the top performing AutoML system in the 2024 AutoMLBenchmark. Nick is co-author of TabPFNMix, the most downloaded tabular foundation model on HuggingFace, and co-author of XTab, one of two tabular foundation model papers published at ICML 2023. He received his master’s degree in Computer Science and Engineering from the University of Minnesota Twin Cities. Starting as a personal competition ML toolkit in 2018, Nick continually expanded the capabilities of AutoGluon and joined Amazon AI in 2019 to open-source the project and work full time on advancing the state-of-the-art in AutoML. Nick has given invited talks and tutorials at the ICML AutoML Workshop (2020-2021), KDD (2020, 2022), GTC (2021), re:Invent (2021, 2024), PyData Seattle (2023), AutoML Conference (2022-2024), and NeurIPS (2022-2024). He has both published in and served as a reviewer at ICML, NeurIPS, and AutoML Conference. Nick is a PC Chair at the AutoML Conference 2025, and co-organized and competed in Kaggle’s AutoML Grand Prix 2024, placing 2nd overall.
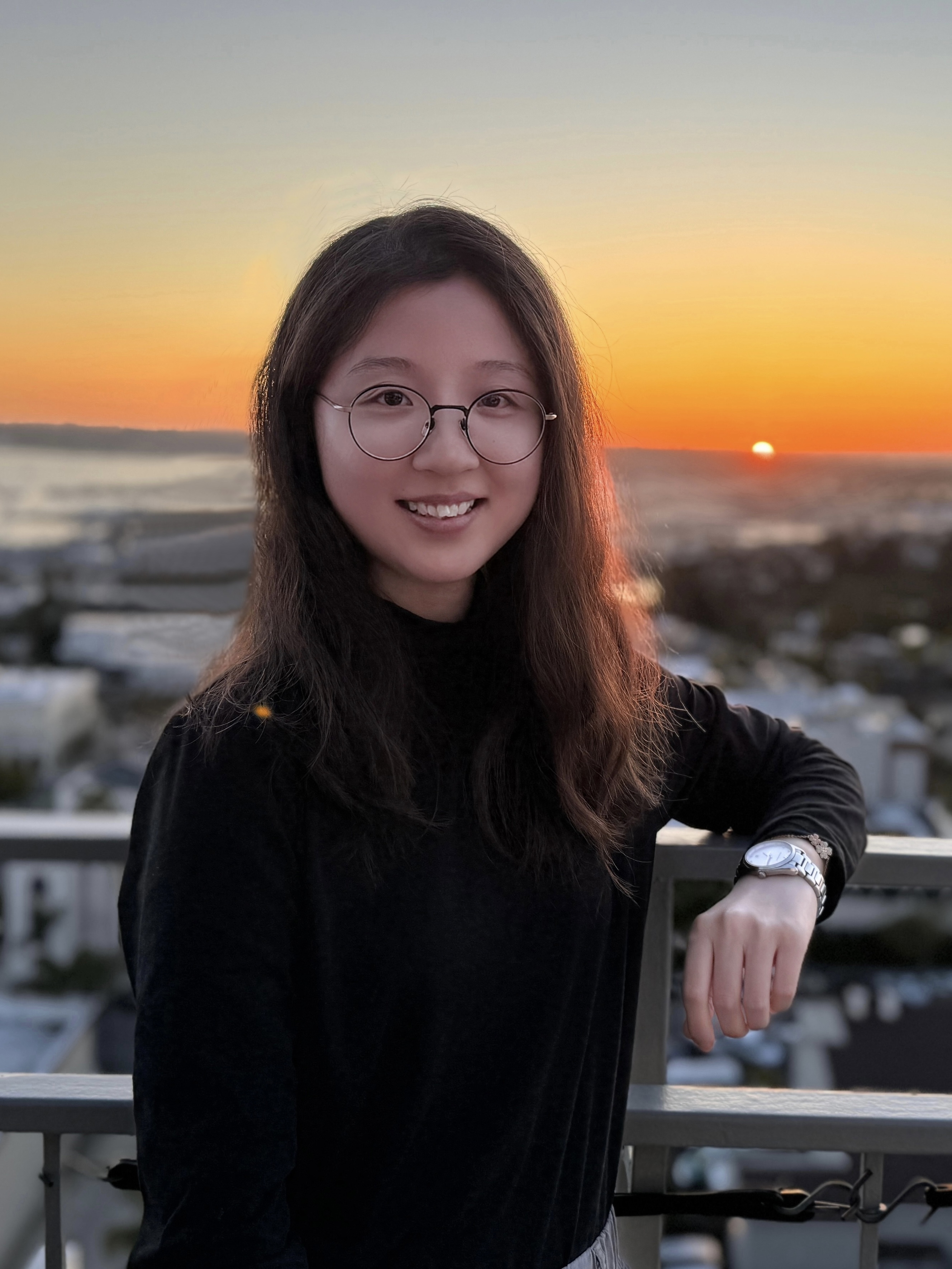
Xiyuan Zhang is an Applied Scientist at Amazon Web Services working on structured data analysis including tabular and time series data. She is the lead author of TabPFNMix, the most downloaded tabular foundation model on HuggingFace, and co-author of Chronos, the most downloaded time series foundation model on HuggingFace. Xiyuan earned her PhD in Computer Science from the University of California, San Diego, advised by Prof. Rajesh K. Gupta and Prof. Jingbo Shang. She has published and received Top Reviewer Award in top machine learning conferences such as NeurIPS. Xiyuan is a recipient of the Qualcomm Innovation Fellowship and has been recognized as a Cyber-Physical-System (CPS) Rising Star. Her broad research interests lie in machine learning for sequential data (time series, spatiotemporal, tabular, NLP), with practical impact including healthcare, IoT, climate science and beyond.
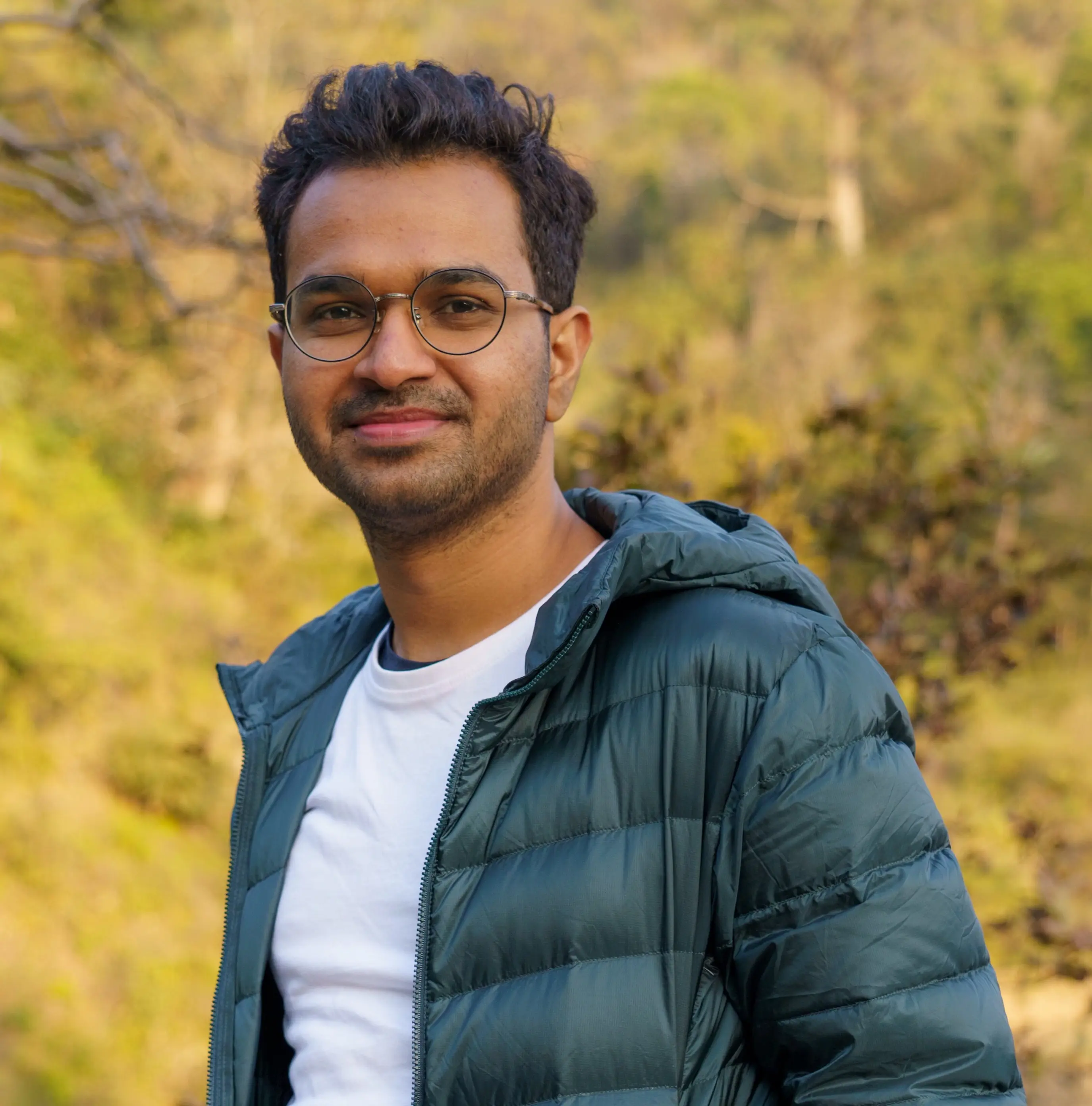
Abdul Fatir Ansari is a Senior Applied Scientist at Amazon Web Services working on time series forecasting. He is the lead developer of the Chronos family of time series foundation models which were amongst the most downloaded models on HuggingFace overall in 2024. He received his PhD in 2022 from the National University of Singapore where he was supervised by Prof. Harold Soh. During his PhD, Abdul Fatir worked on deep generative models for images and time series and was awarded the dean’s award for his PhD research. He has published in and served as a reviewer in top machine learning venues such as ICML, ICLR and NeurIPS, and co-organized the “Time Series in the Age of Large Models” workshop at NeurIPS 2024. His broad research interests lie in the areas of time series analysis and generative modeling, encompassing probabilistic generative modeling, unsupervised learning, and representation learning.
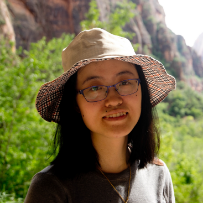
Boran Han is an Applied Scientist at Amazon Web Services working on multimodal time series foundation models and LLM agents for AutoML systems. She is the lead author of AutoGluon Assistant, the only automated agent to score points in Kaggle’s AutoML Grand Prix 2024, placing 10th overall. She earned the Ph.D. degree from Harvard University. She has both published in and served as a reviewer at NeurIPS, ICML, ICLR etc. She has also published in esteemed journals such as Science, Cell, Nature Communications, and Proceedings of the National Academy of Sciences. Her broad research interests lie in multimodal foundation models and its applications in natural science domains such as healthcare and climate.
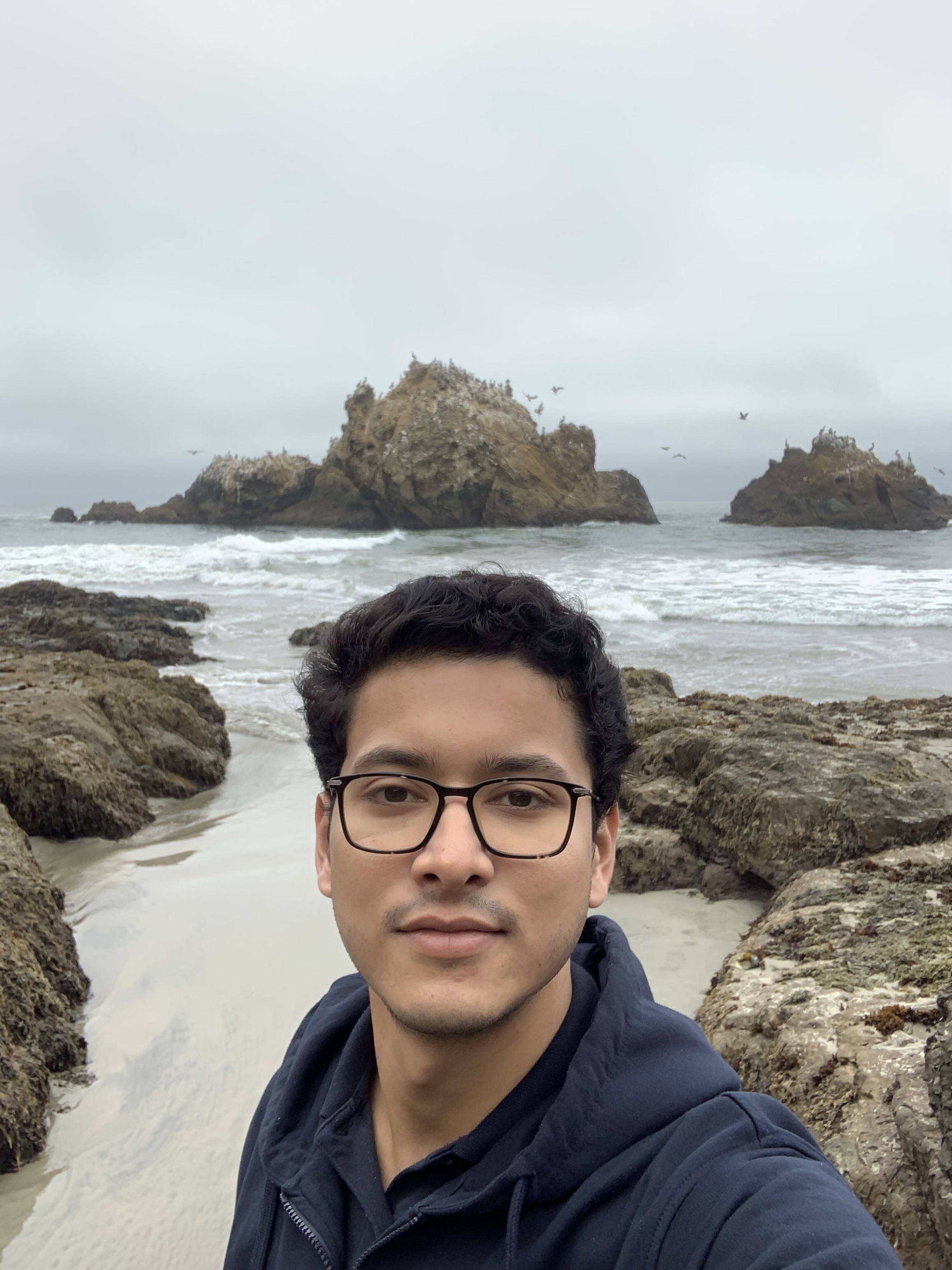
Mononito Goswami is a Ph.D. Candidate at Carnegie Mellon University, advised by Prof. Artur Dubrawski. His research focuses on making foundation models more useful, efficient, and safe, particularly in high-stakes domains like healthcare and education. He led the development of widely used foundation models, including MOMENT (for time series, with over 1M+ downloads on Hugging Face and $2M+ in federal research funding) and IngesTables (for tabular data). His work has been published in top machine learning conferences such as NeurIPS, ICLR, and ICML, where he also serves as a regular reviewer. He was a 2021 Center for Machine Learning and Health (CMLH) fellow and co-organized the highly successful AAAI 2024 Spring Symposium on Clinical Foundation Models. He has also gained industry experience through research roles at Amazon (Applied Scientist Intern) and Google (Student Researcher).
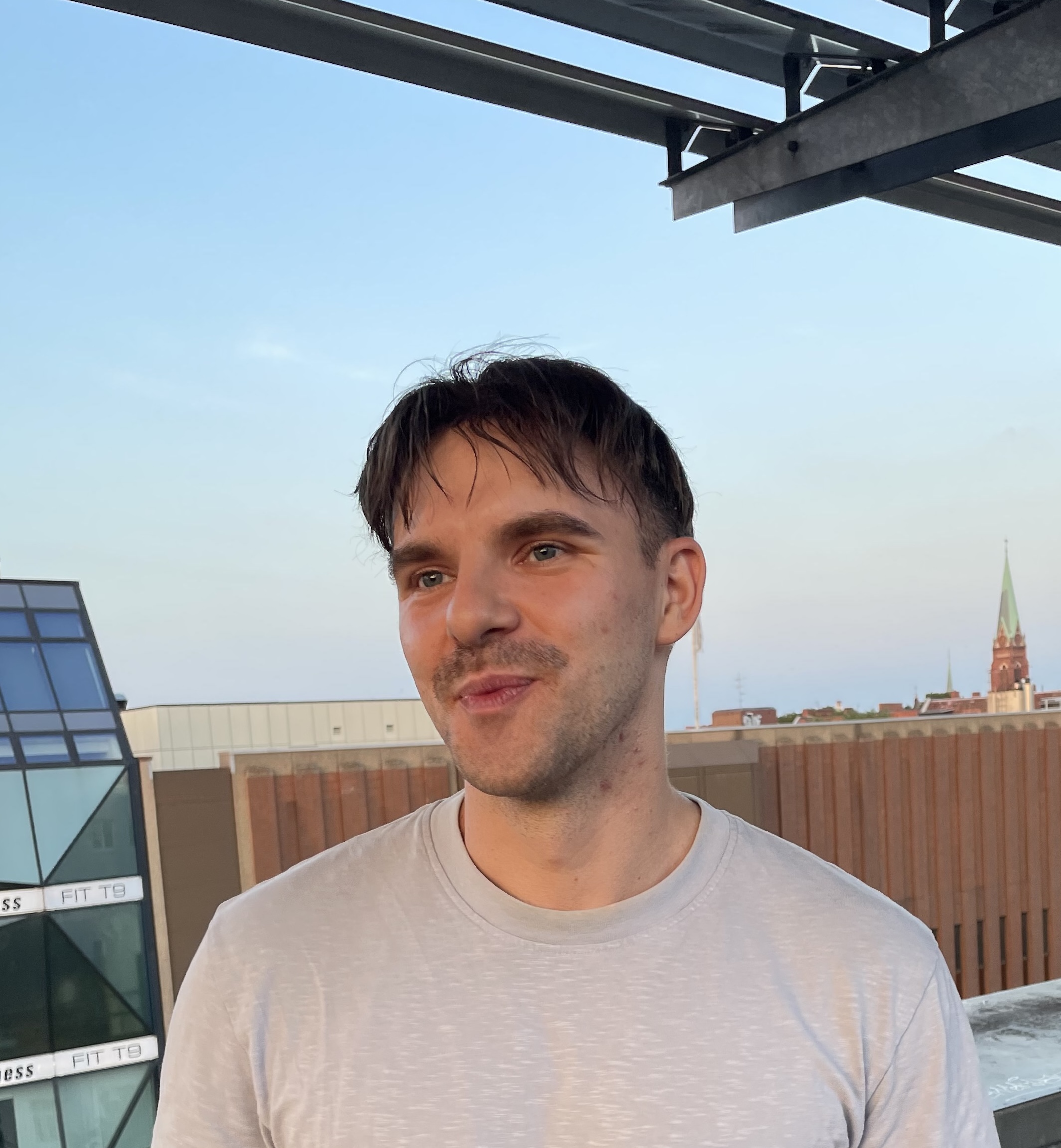
Samuel Müller currently serves as a Postdoc at Meta in New York, where he works in Eytan Bakshy’s research group. During his Ph.D. at the University of Freiburg under Frank Hutter’s supervision, he developed Prior-Data Fitted Networks (PFNs) and co-led the development of TabPFN. His academic journey includes an MPhil from the University of Cambridge and a BSc from ETH Zurich, both in Computer Science. Samuel served as a first author in prestigious venues, including Nature, ICML, ICLR, and NeurIPS. Samuel is especially interested in all kinds of pre-training techniques on artificial, randomly-sampled data.
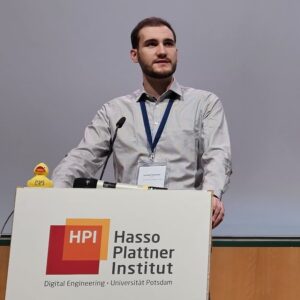
Lennart Purucker is a Ph.D. student at the University of Freiburg, supervised by Prof. Frank Hutter. Before his Ph.D., Lennart completed his M.Sc. in Computer Science at RWTH Aachen. He researches automated machine learning, large language models, and foundation models for (small) tabular data. Lennart is actively involved in the research community, reviewing for various conferences, serving as the Reproducibility Chair for the AutoML Conference (2023–2025), co-organizing the AutoML Seminar, and contributing to open science as a core member of OpenML. Lennart actively collaborates with interdisciplinary researchers as part of the Small Data Initiative and industry partners through the University of Freiburg. He co-authored the latest version of TabPFN, published in Nature, a foundation model for predictive tasks on small tabular data. Furthermore, Lennart is a developer of AutoGluon, a popular AutoML system for tabular data. In 2024, he led the AutoML Grandmasters team in Kaggle’s AutoML Grand Prix for tabular data, employing AutoGluon and TabPFN in highly competitive competitions to secure second place.
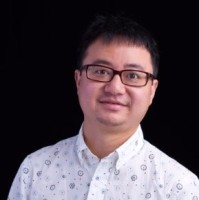
Yuyang (Bernie) Wang is a Principal Machine Learning Scientist in Amazon AI Labs, working mainly on large-scale probabilistic machine learning with its application in Forecasting. He received his PhD in Computer Science from Tufts University, MA, US and he holds an MS from the Department of Computer Science at Tsinghua University, Beijing, China. His research interests span statistical machine learning, numerical linear algebra, and random matrix theory. In forecasting, Yuyang has worked on all aspects ranging from practical applications to theoretical foundations.
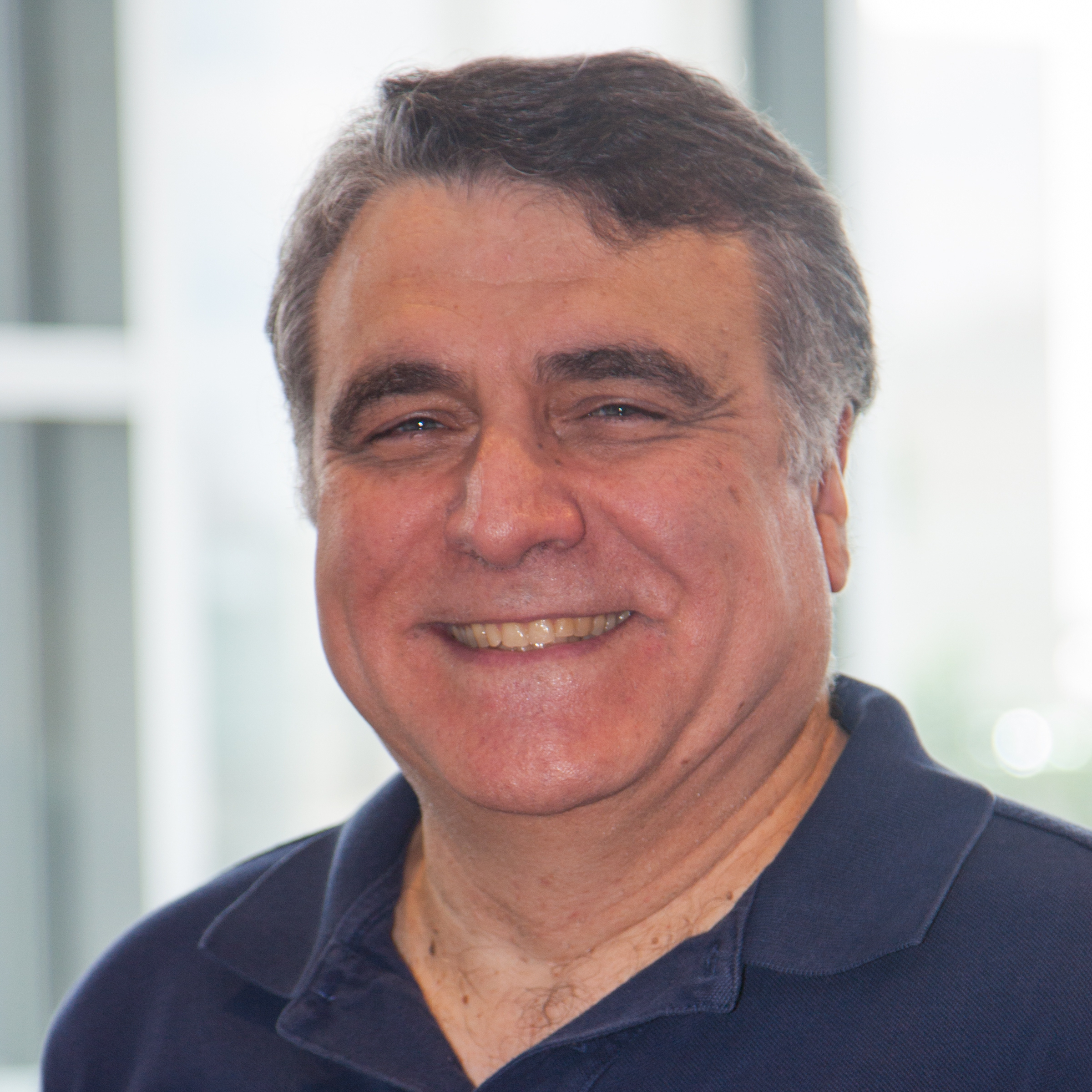
Carnegie Mellon University
Pittsburgh (US)
Christos Faloutsos is a Professor at Carnegie Mellon University and an Amazon Scholar. He is the recipient of the Fredkin Professorship in Artificial Intelligence (2020); he has received the Presidential Young Investigator Award by the National Science Foundation (1989), the Research Contributions Award in ICDM 2006, the SIGKDD Innovations Award (2010), the PAKDD Distinguished Contributions Award (2018), 32 “best paper” awards (including 8 “test of time” awards), and four teaching awards. Eight of his advisees or co-advisees have attracted KDD or SCS dissertation awards. He is an ACM Fellow and has served as a member of the executive committee of SIGKDD; he has published over 500 refereed articles, 17 book chapters and three monographs. He holds 12 patents (and several more are pending), and he has given over 50 tutorials and over 25 invited distinguished lectures. His research interests include large-scale data mining with emphasis on graphs and time sequences; anomaly detection, tensors, and fractals.
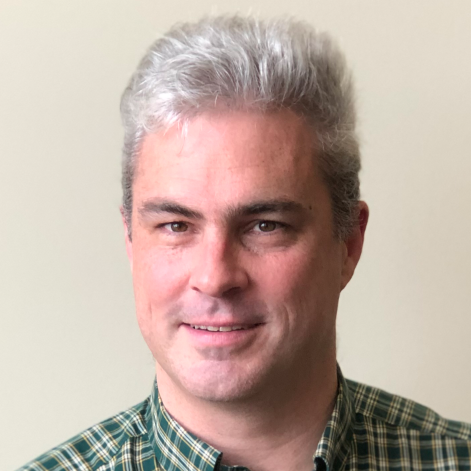
Michael Mahoney is at the University of California at Berkeley in the Department of Statistics and at the International Computer Science Institute (ICSI). He is also an Amazon Scholar as well as head of the Machine Learning and Analytics Group at the Lawrence Berkeley National Laboratory. He works on algorithmic and statistical aspects of modern large-scale data analysis. Much of his recent research has focused on large-scale machine learning, including randomized matrix algorithms and randomized numerical linear algebra, scientific machine learning, scalable stochastic optimization, geometric network analysis tools for structure extraction in large informatics graphs, scalable implicit regularization methods, computational methods for neural network analysis, physics informed machine learning, and applications in genetics, astronomy, medical imaging, social network analysis, and internet data analysis. He received his PhD from Yale University with a dissertation in computational statistical mechanics, and he has worked and taught at Yale University in the mathematics department, at Yahoo Research, and at Stanford University in the mathematics department. Among other things, he was on the national advisory committee of the Statistical and Applied Mathematical Sciences Institute (SAMSI), he was on the National Research Council’s Committee on the Analysis of Massive Data, he co-organized the Simons Institute’s fall 2013 and 2018 programs on the foundations of data science, he ran the Park City Mathematics Institute’s 2016 PCMI Summer Session on The Mathematics of Data, he ran the biennial MMDS Workshops on Algorithms for Modern Massive Data Sets, and he was the Director of the NSF/TRIPODS-funded FODA (Foundations of Data Analysis) Institute at UC Berkeley.
Reviewer Acknowledgement
We would like to sincerely thank all the reviewers who generously contributed their time and expertise to support our workshop. Your thoughtful feedback, careful evaluations, and constructive suggestions were essential in ensuring the quality and impact of the accepted submissions. We are deeply grateful for your commitment to fostering meaningful research and discussion in this growing community!
Dan Rosenbaum, Ege Onur Taga, Felix den Breejen, Herilalaina Rakotoarison, John F Bronskill, Yunkai Zhang, Yinghao Zhu, Liang Sun, Michał Wiliński, Sophia Huiwen Sun, Yifu Cai, Anthony E Culos, Arjun Ashok, Ziyang Song, Andrei Margeloiu, Vinayak Gupta, Zelin He, Sebastian Pineda Arango, Zhe Li, Andrej Tschalzev, Arian Khorasani, Matthias Feurer, Arjun Choudhry, Marcel Kollovieh, Defu Cao, Lorenzo Stella, Mykhailo Koshil, Ivan Rubachev, Young-Jin Park, Gerald Woo, Azul Garza, Maximilian Schambach, Boje Deforce, Yury Gorishniy, Vincent Zhihao Zheng, Chenghao Liu, Andres Potapczynski, Junwei Ma, Sunita Sarawagi, Oleksandr Shchur, Ching Chang, Kijung Yoon, Peisong Niu, Afrin Dange, Valentin Thomas, Sean Bin Yang, Ehsan Hoseinzade, Yunfei Luo, David Holzmüller, Abhilash Neog, Divij Gupta, Andreas C Mueller, Andrew Robert Williams, Aaron Klein, Yingcong Li, Hao Zhao, Ranak Roy Chowdhury, Nikolaos Evangelou, Pengwei Li, Matthias Hertel, Payal Mohapatra, Sadhika Malladi, Jiuding Duan, Jingang QU, Taeyoung Kim, Andreas Auer, Bhargav Jairam Shetgaonkar, Haibei Zhu, Yajun Liu, Sai Prasanna Teja Reddy Bogireddy, Ajay Nagrale, Phanindra Reddy Madduru, Peng Andy, Joachim Fainberg, Tejas Anvekar, Neta Rabin, Mateusz Koren, Yihao Ang, Ali Nawaz, Vedant Sanil